Our innovation and technologies
Key Technology
A portfolio of AI-augmented technologies and state-of-the-art algorithms (similar to technologies Google uses to identify handwriting), empower the evaluation of billions of molecules to design drugs faster and cheaper.
Target SpaceTM
A database of 160’000 targets as 3D structures
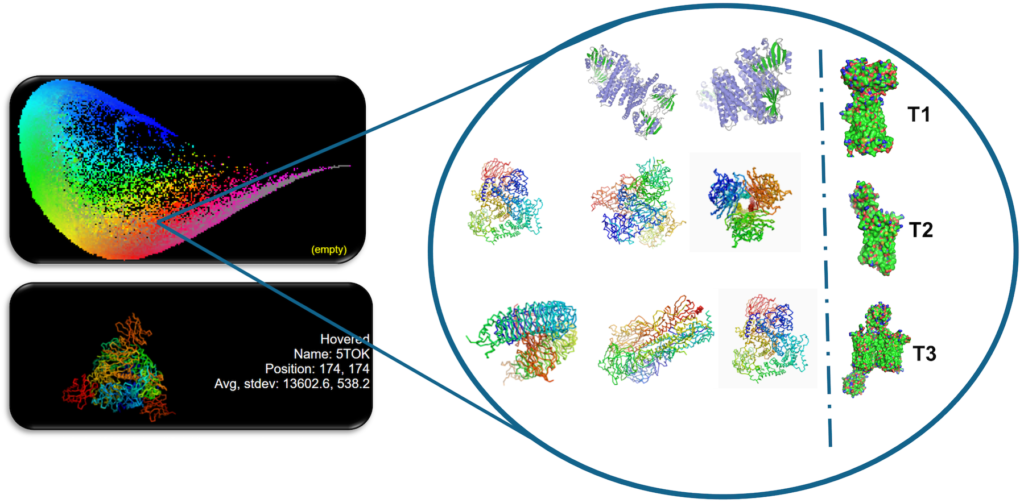
Target Deep analysis
GDBspace first scan reported co-crystallized 3D structures to obtain 2D scans of the protein with its ligand bound to it, then perform 3D reconstruction from these 2D scans, leading to the precise identification of the target binding sites.
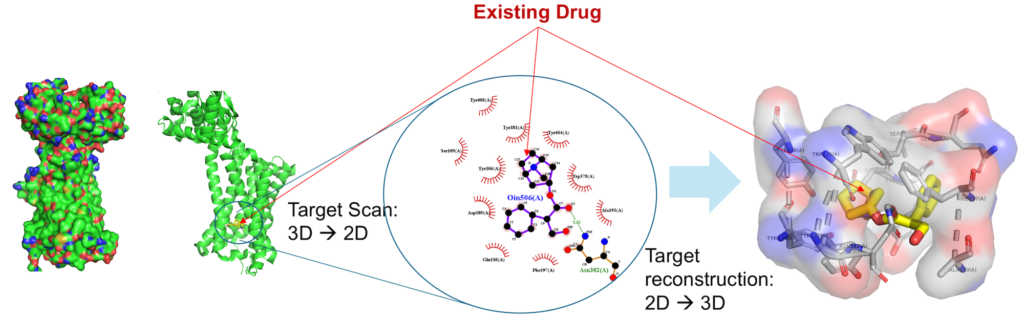
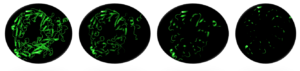
Selected Publications:
- Xian Jin et al., “PDB-Explorer: a web-based interactive map of the protein data bank in shape space”, BMC Bioinformatics, 2015.
- Mahendra Awale, et al., “MQN-Mapplet: Visualization of chemical space with interactive maps of DrugBank, ChemBL, PubChem, GDB-11, and GDB-13, J. Chem. Inf. Model., 2013
PepSpaceTM Peptides Virtual Library
GDBspace enumerates more than 10 billion peptide and peptidomimetic peptide library using proprietary fingerprint uniquely applied to any modalities (peptides, small molecules, oligonucleotides).
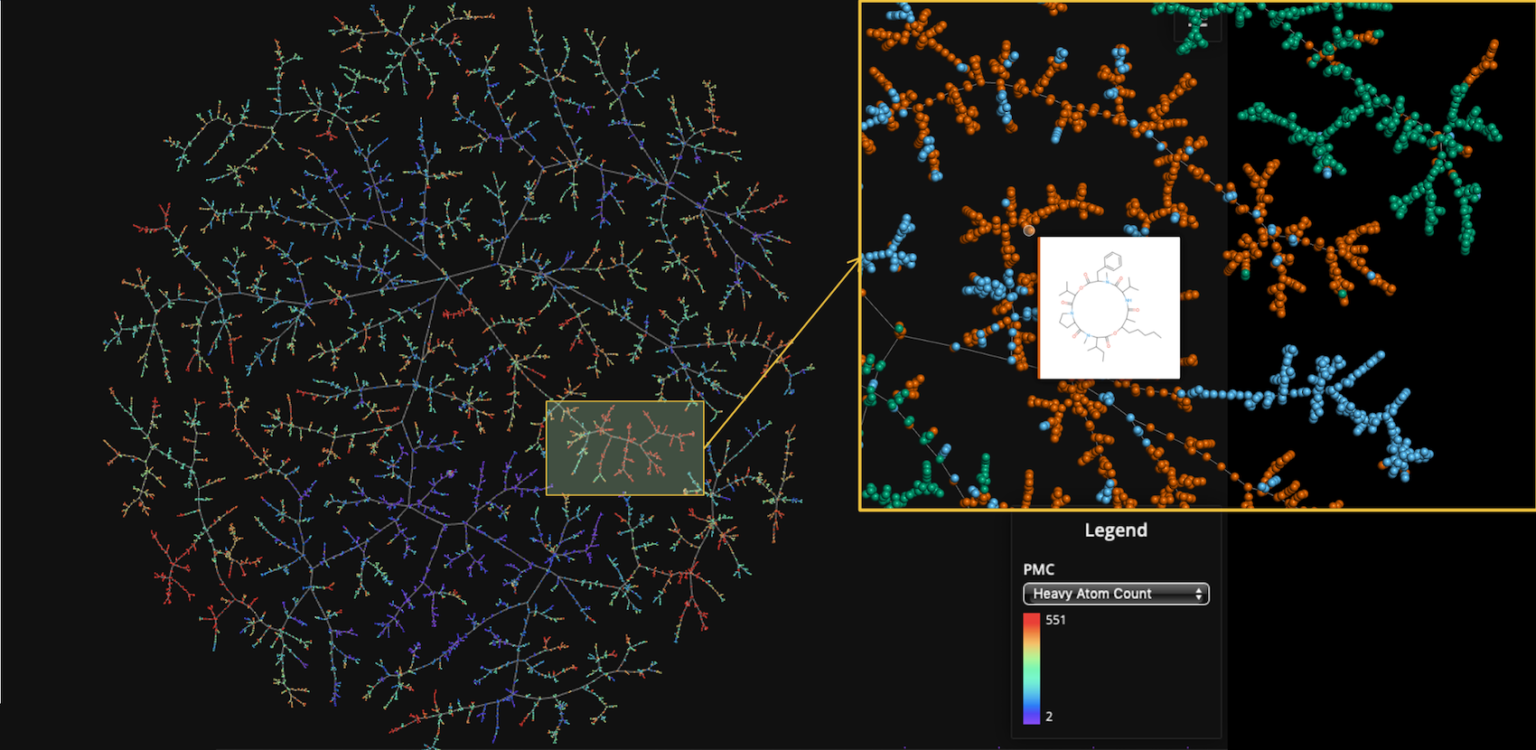
Extraction by Fingerprint and Virtual Screening
Focused screening libraries specifically designed to each project specificity are screened, followed by multiparameter optimization of the potential candidate. As a last step, binding of the compounds into the different desired targets using docking are done to select and confirm the selected molecules. At least 3 rounds of iteration are performed by GDBspace, ensuring the selection of only the most relevant compounds for biological validation.
Multiparameter Optimization:
- Secondary/Tertiary structure
- Activity
- Presence of aromatic and positive residues
- Toxicity
- Potential hydrogen bonds
- Length
- Half-life
- Amphiphilicity
- LogP
- Molecular weight
- Isoelectric point
- Nature of N- and C-termini
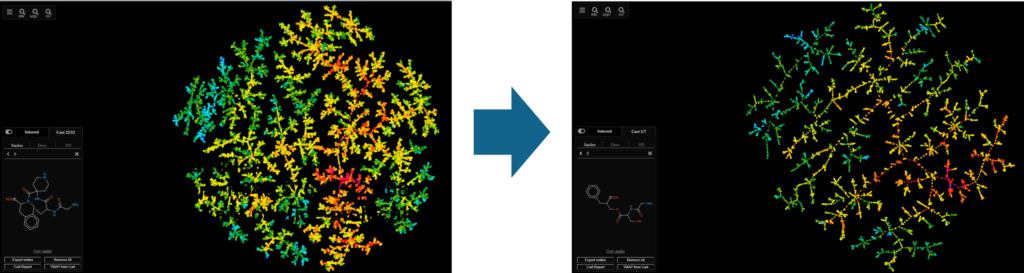
Selected Publications:
- Alice Capecchi and Jean-Louis Reymond, “Peptides in chemical space”, Medicine in Drug Discovery, 2021
- Alice Capecchi, Daniel Probst and Jean-Louis Reymond, “One molecular fingerprint to rule them all: drugs, biomolecules and the metabolome”, Cheminform., 2020
- Daniel Probst et al., “Visualization of very large high-dimensional datasets as minimum spanning trees”, J. Cheminform., 2020.
- Daniel Probst and Jean-Louis Reymond, “FUn: a framework for interactive visualizations of large, high-dimensional datasets on the web”, Bioinformatics, 2018.
- Jean-Louis Reymond et al., “Exploring chemical space for drug discovery using the chemical universe database”, American Chemical Society Chemical Neuroscience, 2012.